
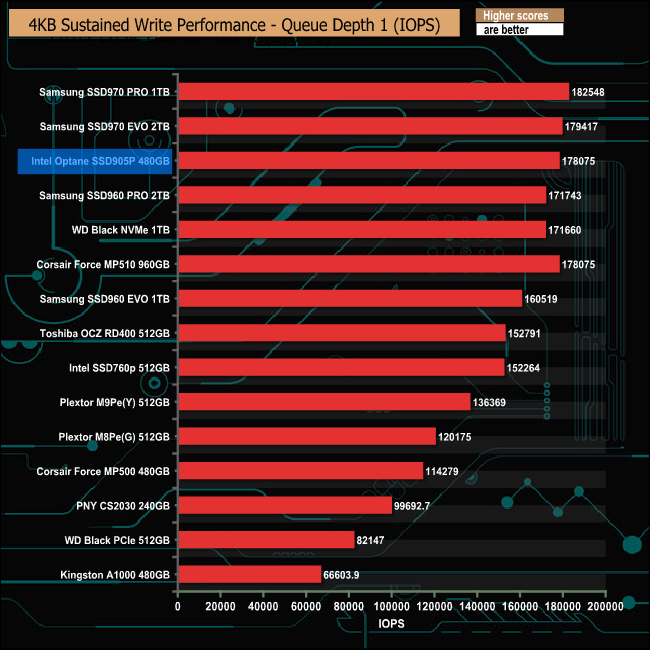

For example, this approach has been used to identify variants associated with patterns shared across multiple MetS-related traits however, this approach has several limitations. To confront the challenges posed by the analysis of systems of many traits, some authors considered using phenotype-derived principal components (PCs) as traits in GWA analyses. These challenges get exacerbated by the fact that whole-genome scans require performing these tests for potentially millions of variants and by the very large sample size of modern biobanks. This exponential growth in the number of hypotheses that need to be tested creates obvious computational difficulties, makes type I error control challenging, and renders interpretation and communication of results difficult. The number of possible pleiotropic configurations grows exponentially with the number of traits for example, in a system of 10 traits there are 1013 configurations which involve the same variant having effects in at least two traits. For example, in a system of three traits, there are four possible configurations involving pleiotropy: one for associations with three traits and three involving two traits. However, mapping these loci on multiple traits can be computationally and statistically challenging because of the large number of tests involved. Therefore, in recent years, there has been an increased interest in mapping genetic loci with pleiotropic effects.
SEQUENTIAL TESTING AT INTEL DRIVER
Evidence from quantitative genetic studies and from genome-wide association (GWA) analyses suggest that pleiotropy (i.e., variants with simultaneous effects on several traits) is an important driver of the genetic correlation between comorbid conditions. Many human diseases (e.g., hypertension, gout, and diabetes) cluster into syndromes. Our results confirm previously reported loci and lead to several novel discoveries that link MetS-related traits through plausible biological pathways. We validate our results using previous studies documented in the GWAS-catalog and using data from GTEx. We found abundant pleiotropy and report 170, 44, and 18 genomic regions harboring SNPs with pleiotropic effects in at least two, three, and four of the seven traits, respectively. To demonstrate the use of the methods and software, we present a whole-genome scan in search of loci with pleiotropic effects on seven traits related to metabolic syndrome (MetS) using UK-Biobank data (n~300 K distantly related white European participants).
SEQUENTIAL TESTING AT INTEL SOFTWARE
Using simulations, we show that the methods implemented in the software are powerful and have adequate type-I error rate control. In this study, we present a sequential test and software that can be used to test pleiotropy in large systems of traits with biobank-sized data.

This has limited the adoption of sequential testing for pleiotropy mapping at large scale. However, the existing methods and the available software do not scale to analyses involving millions of SNPs and large datasets. Sequential testing is a powerful approach for mapping genes with pleiotropic effects. Identifying variants with pleiotropic effects on multiple health-related traits can improve the biological understanding of gene action and disease etiology, and can help to advance disease-risk prediction. Pleiotropy (i.e., genes with effects on multiple traits) leads to genetic correlations between traits and contributes to the development of many syndromes.
